Aberration Separation
Revealing the preference of deep-learning model on optical aberrations
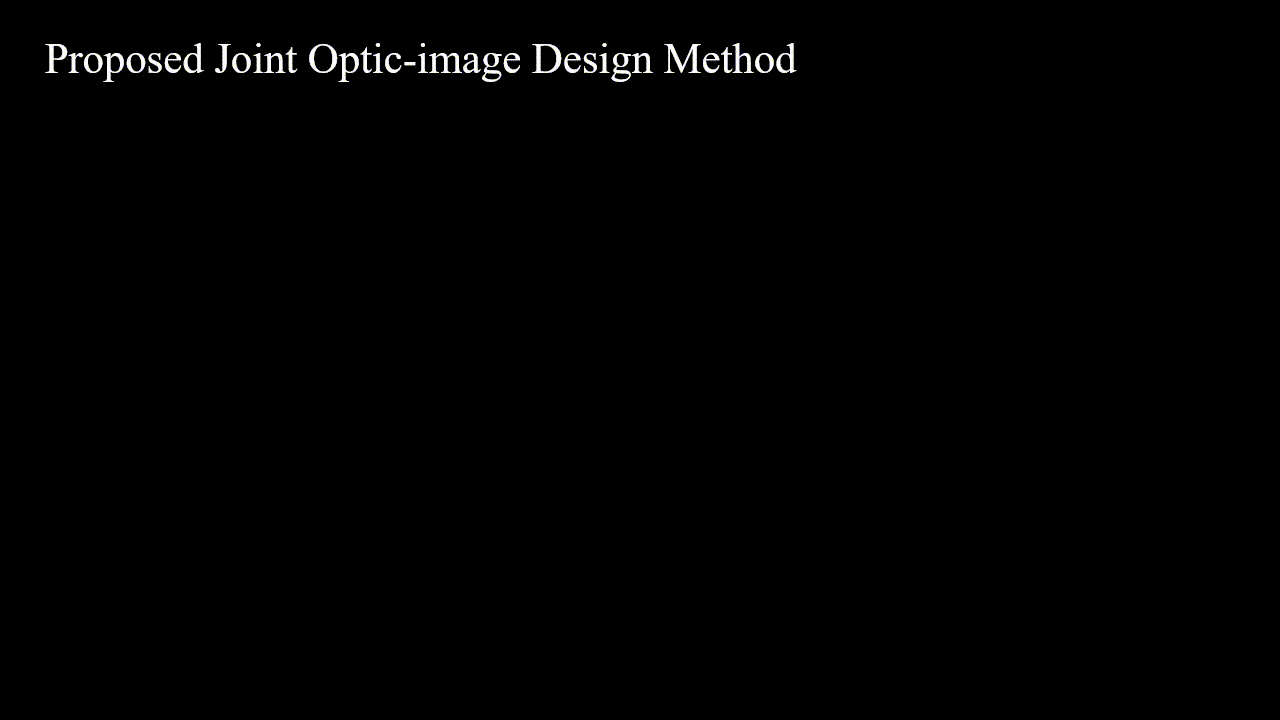
Abstract:
The joint design of the optical system and the downstream algorithm is a challenging and promising task. Due to the demand for balancing the global optima of imaging systems and the computational cost of physical simulation, existing methods cannot achieve efficient joint design of complex systems such as smartphones and drones. In this work, starting from the perspective of the optical design, we characterize the optics with separated aberrations. Additionally, to bridge the hardware and software without gradients, an image simulation system is presented to reproduce the genuine imaging procedure of lenses with large field-of-views. As for aberration correction, we propose a network to perceive and correct the spatially varying aberrations and validate its superiority over state-of-the-art methods. Comprehensive experiments reveal that the preference for correcting separated aberrations in joint design is as follows: longitudinal chromatic aberration, lateral chromatic aberration, spherical aberration, field curvature, and coma, with astigmatism coming last. Drawing from the preference, a 10% reduction in the total track length of the consumer-level mobile phone lens module is accomplished. Moreover, this procedure spares more space for manufacturing deviations, realizing extreme-quality enhancement of computational photography. The optimization paradigm provides innovative insight into the practical joint design of sophisticated optical systems and post-processing algorithms.
Background:
With the popularity of mobile photography (e.g., smartphones, action cameras, drones, etc.), the optics of cameras tend to be more miniaturized and lightweight for imaging. The engaged methods can be divided into several categories: 1. from the perspective of image processing and 2. from the consideration of the whole system with end-to-end optimization. While they all have some imitations in implementation. So what if we start from the perspective of the optical design?
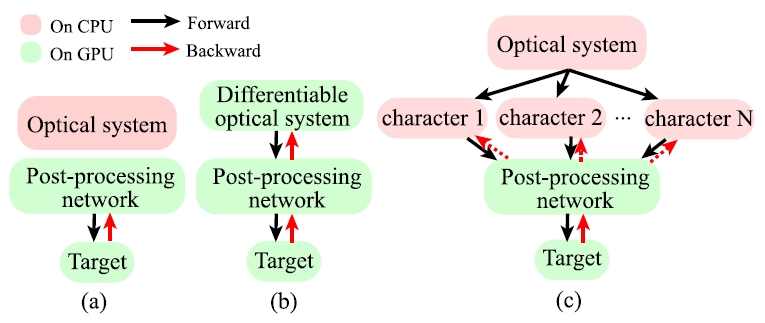
Method:
Our aim is to investigate the preference for correcting separated aberrations in joint optic-image design.
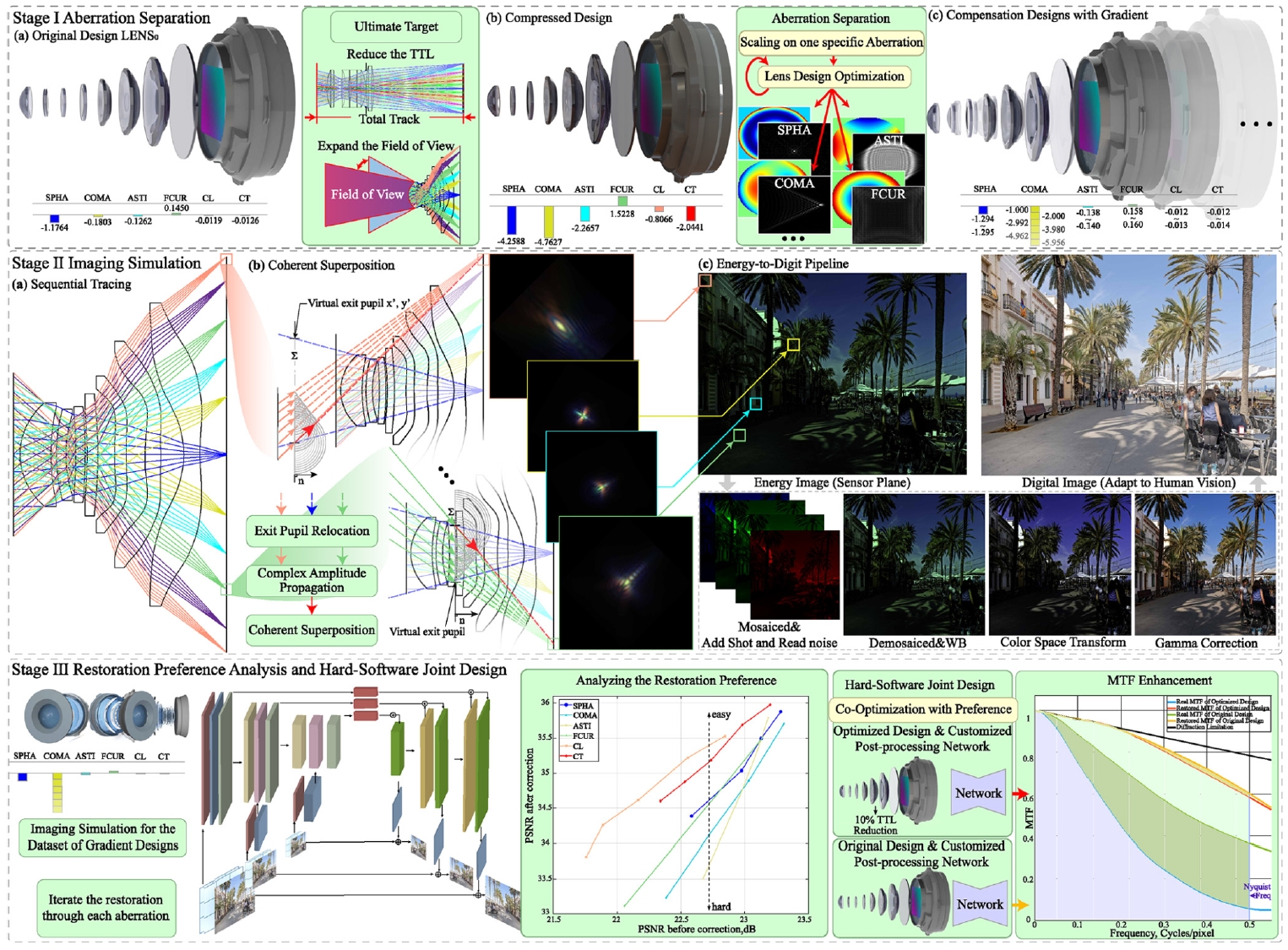
Compound lens design has various degrees of freedom, and traversing all the potential designs is time-consuming. Following the vector quantization in auto-regressive, we note that mapping intricate data (e.g., lens configuration) to a highdimensional representation (e.g., aberrations coefficients) could realize a more comprehensive characterization. Fortunately, imaging quality is mostly corrupted by the degradation of primary aberrations, which happens to be quantified by Seidel coefficients. Therefore, considering the trade-off between accuracy and concision, Seidel is a better option in the view of aberration decomposition. Here we show the design results of our aberration separation strategy
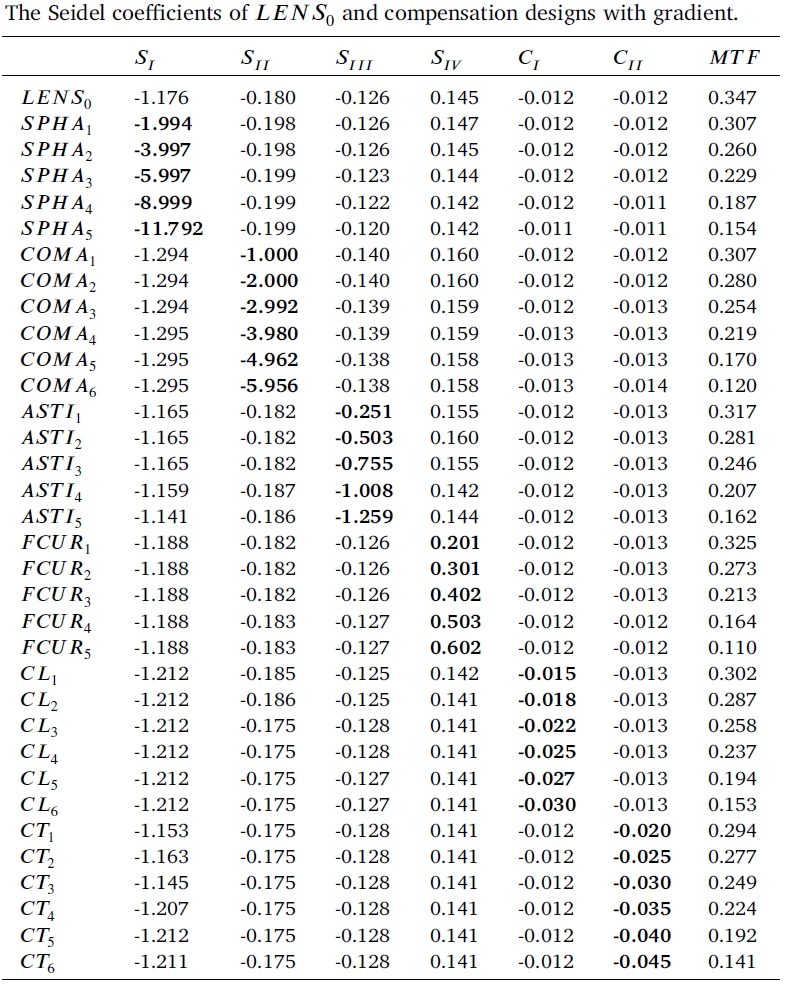
And we analysis the distribution of degradation across all designs. This results shows that our strategy could obtain adquately sampled aberration/degration space.
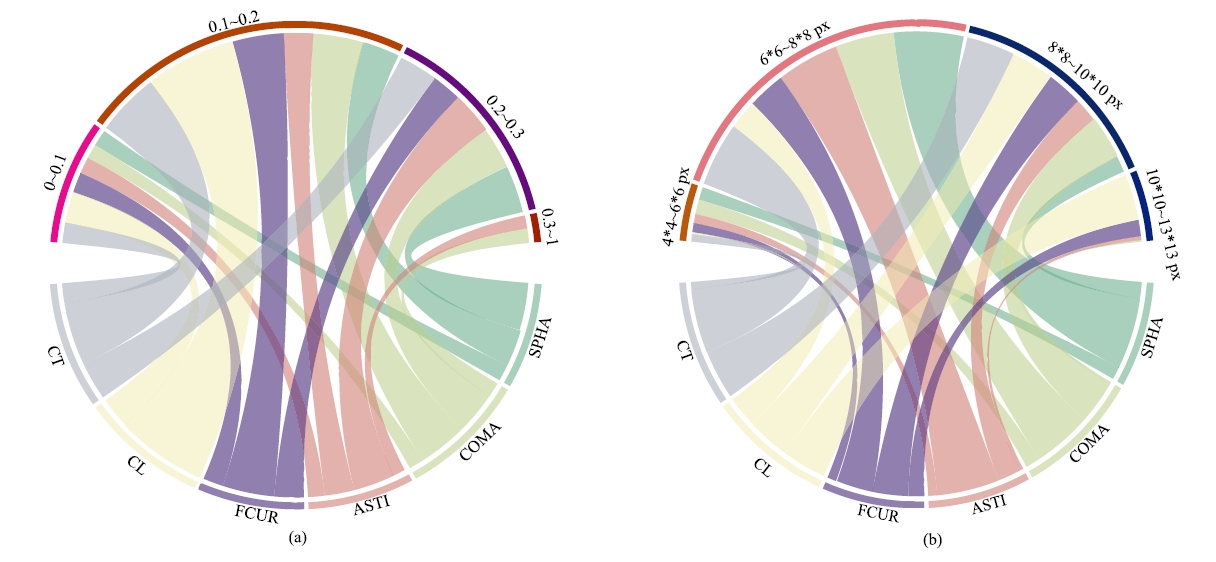
By the design strategy of aberration separation, we work with the same restoration pipeline used in Imaging Simulation. In this way, we could analyse which aberrations are easier for correction, and which aberrations are more difficult to restore.
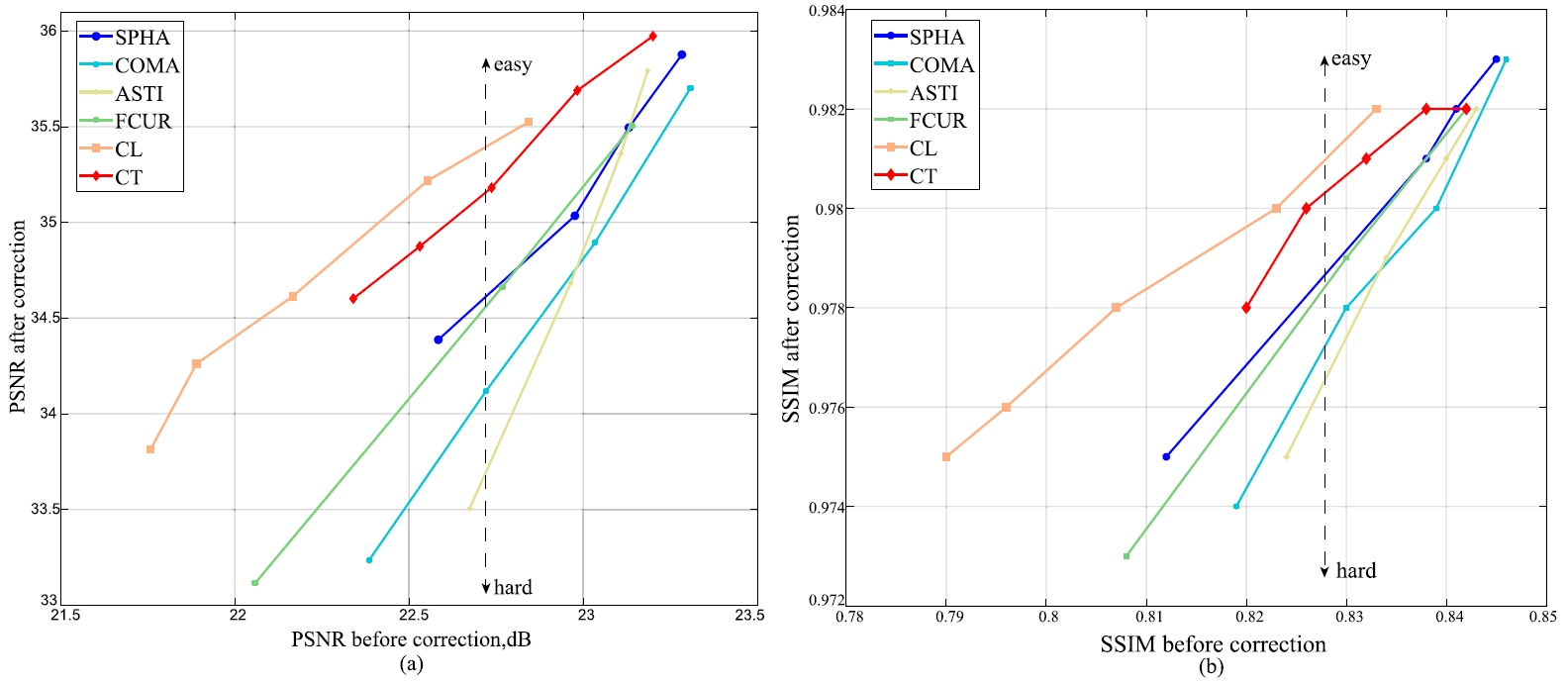
From the results above, we can easily find out that the deep learning model has a preference to different optical aberrations. The sequence is given as: CL > CT > SPHA > FCUR > COMA > ASTI (larger means easier to correct).
After getting this prior, we start to use this as a guidance for photography object lens design. And we realize the optimization of 10% height decrease in the main camera of mobile phone (from 6.1mm to 5.5mm).
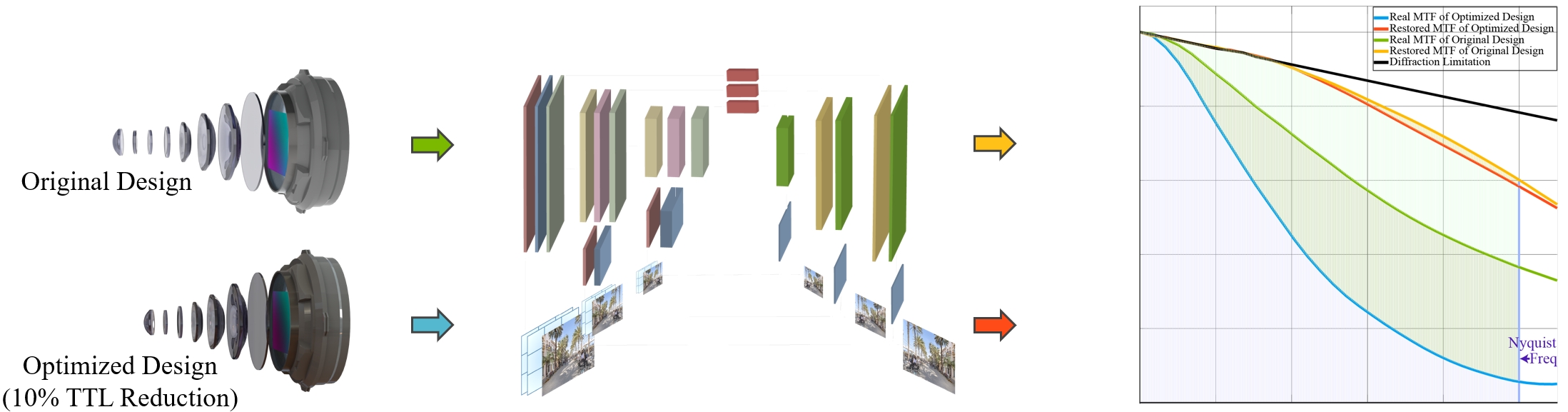
We do the same analysis procedures on two different objective lens configuration, and the conclusions are the same!
Our Main Observation:
What optical aberrations do you need to control first in optical designing?
Follow me and say ASTI > COMA > FCUR > SPHA > CT > CL (loudly)
Let this MANTRA makes your LENS DESIGN BETTER!!!