Tolerance Modeling
how to predict the error in mass production?
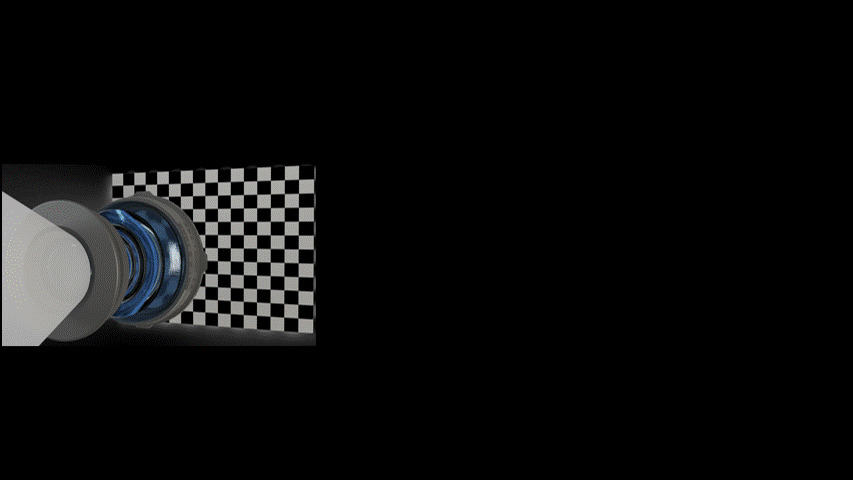
Abstract:
Correcting the optical aberrations and the manufacturing deviations of cameras is a challenging task. Due to the limitation on volume and the demand for mass production, existing mobile terminals cannot rectify optical degradation. In this work, we systematically construct the perturbed lens system model to illustrate the relationship between the deviated system parameters and the spatial frequency response (SFR) measured from photographs. To further address this issue, an optimization framework is proposed based on this model to build proxy cameras from the machining samples’ SFRs. Engaging with the proxy cameras, we synthetic data pairs, which encode the optical aberrations and the random manufacturing biases, for training the learning-based algorithms. In correcting aberration, although promising results have been shown recently with convolutional neural networks, they are hard to generalize to stochastic machining biases. Therefore, we propose a dilated Omni-dimensional dynamic convolution (DOConv) and implement it in post-processing to account for the manufacturing degradation. Extensive experiments which evaluate multiple samples of two representative devices demonstrate that the proposed optimization framework accurately constructs the proxy camera. And the dynamic processing model is well-adapted to manufacturing deviations of different cameras, realizing perfect computational photography. The evaluation shows that the proposed method bridges the gap between optical design, system machining, and postprocessing pipeline, shedding light on the joint of image signal reception (lens and sensor) and image signal processing (ISP).
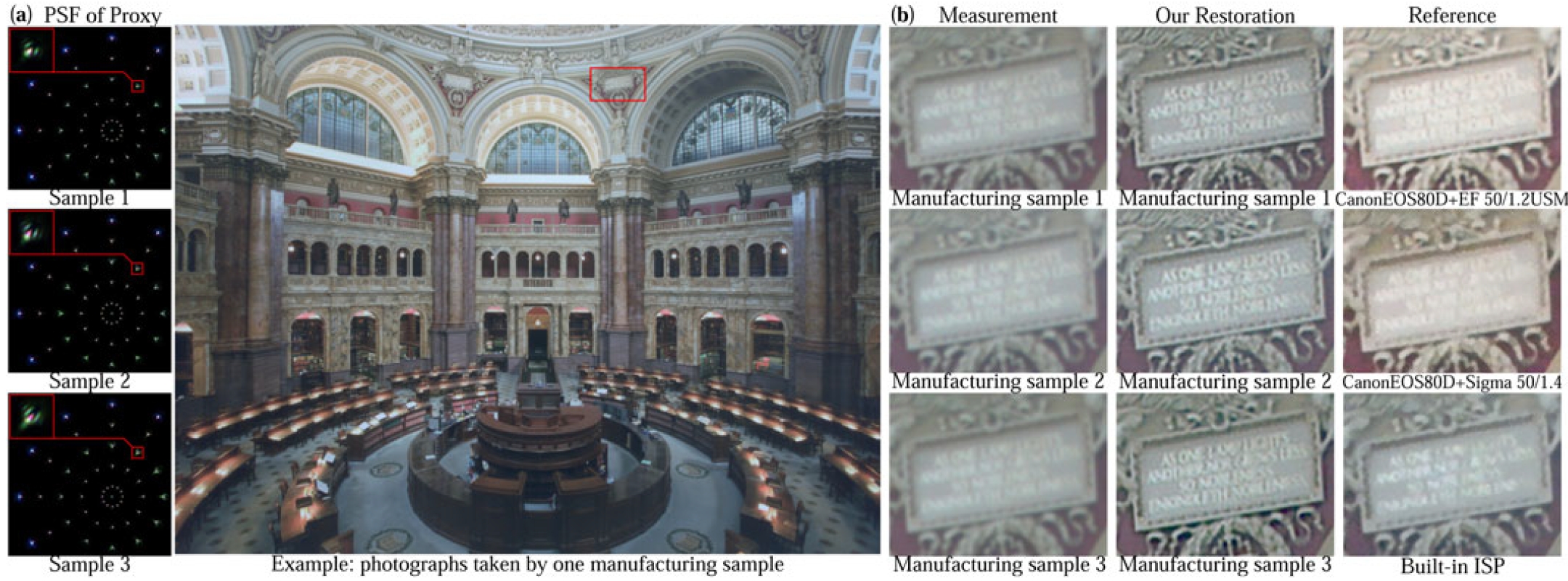
Background:
In the machining and assembly procedure of imaging systems, deflection and manufacturing bias affect the shape and positions of lenses. Even subtle shape or position variations will introduce additional aberrations, which significantly degrade the optical performance of cameras. To be more specific, the deflection will lead to the point spread function (PSF) differenceof the symmetrical field-of-view (FoV), and the manufacturing deviation will cause the overall decrease in SFR. Hence, analyzing the biases between the ideal and manufacturing is a critical issue in the optomechanical design of the imaging system, and it is essential for improving processing quality and controlling the cost.
Method:
We built the physical-based camera perturbation model to predict the deviation of systems, aiming at constructing proxy cameras whose imaging results are close to reality.
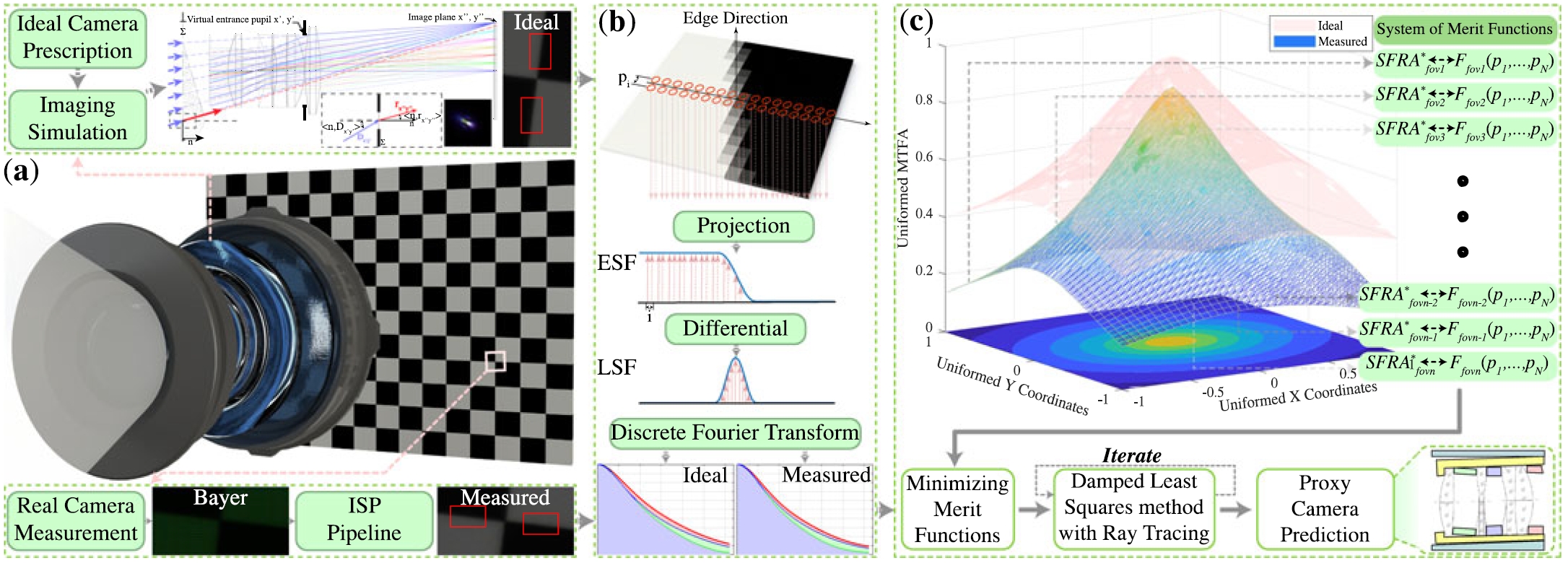
The procedure of the turbulance prediction is just like the procedure of optical system optimization. Different from the traditional optimization used in Zemax or CodeV, this framework consider the sampling of sensor plane and the simulated with a comprehensive imaging pipeline.
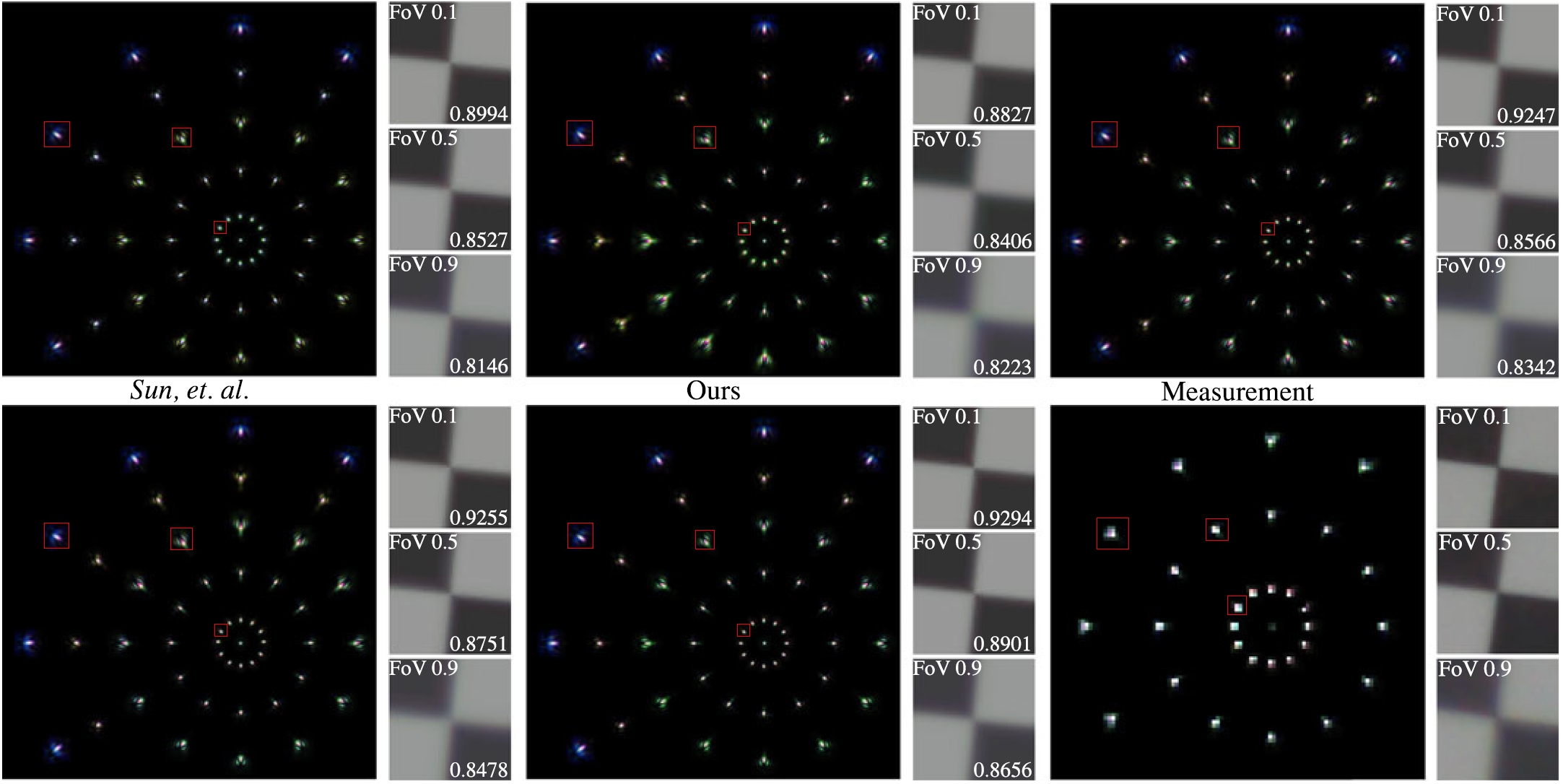
the proposed perturbation model outperforms optical design program (e.g., CODE V) and other SOTA algorithms. The proposed dynamic post-processing pipeline shed light on the joint of image signal reception (lens and sensor) and image signal processing (ISP).
For training the restoring network, we use proxy cameras to generate the data pairs that characterize the mapping of optical degradation. To be specific, we sample many real device to get the range of tolerance, and construct a large dataset for modeling the cameras in mass production. Then, we propose two deep learning models for correction. One model is blind and another is non-blind. The correction results show that simulating mass production could realize minimal computational cost and fast adapting to the data acquisition of new devices with tolerance.
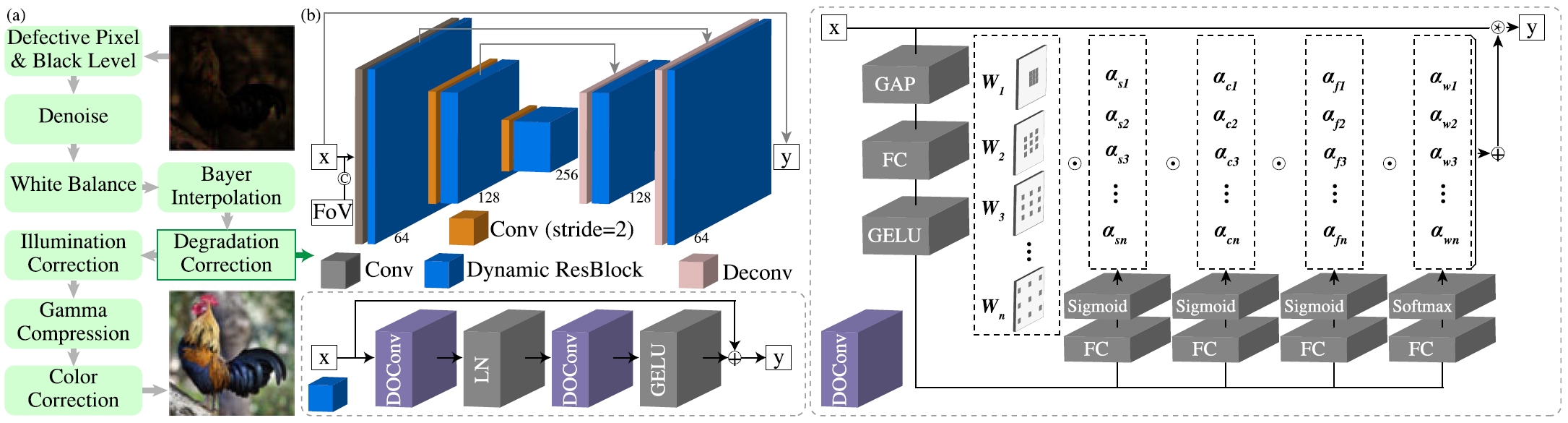
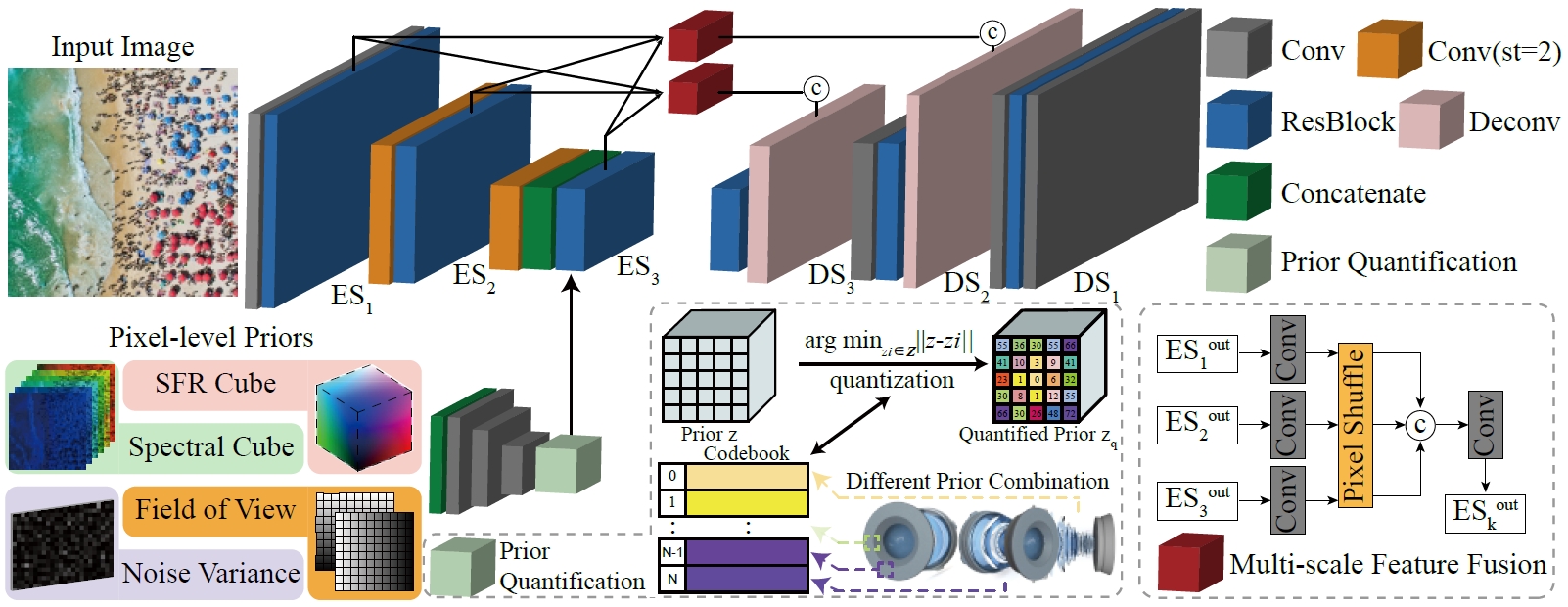
Experiments:
We show some visualization here, for detailed comparisons and illustrations, please refer to our paper.
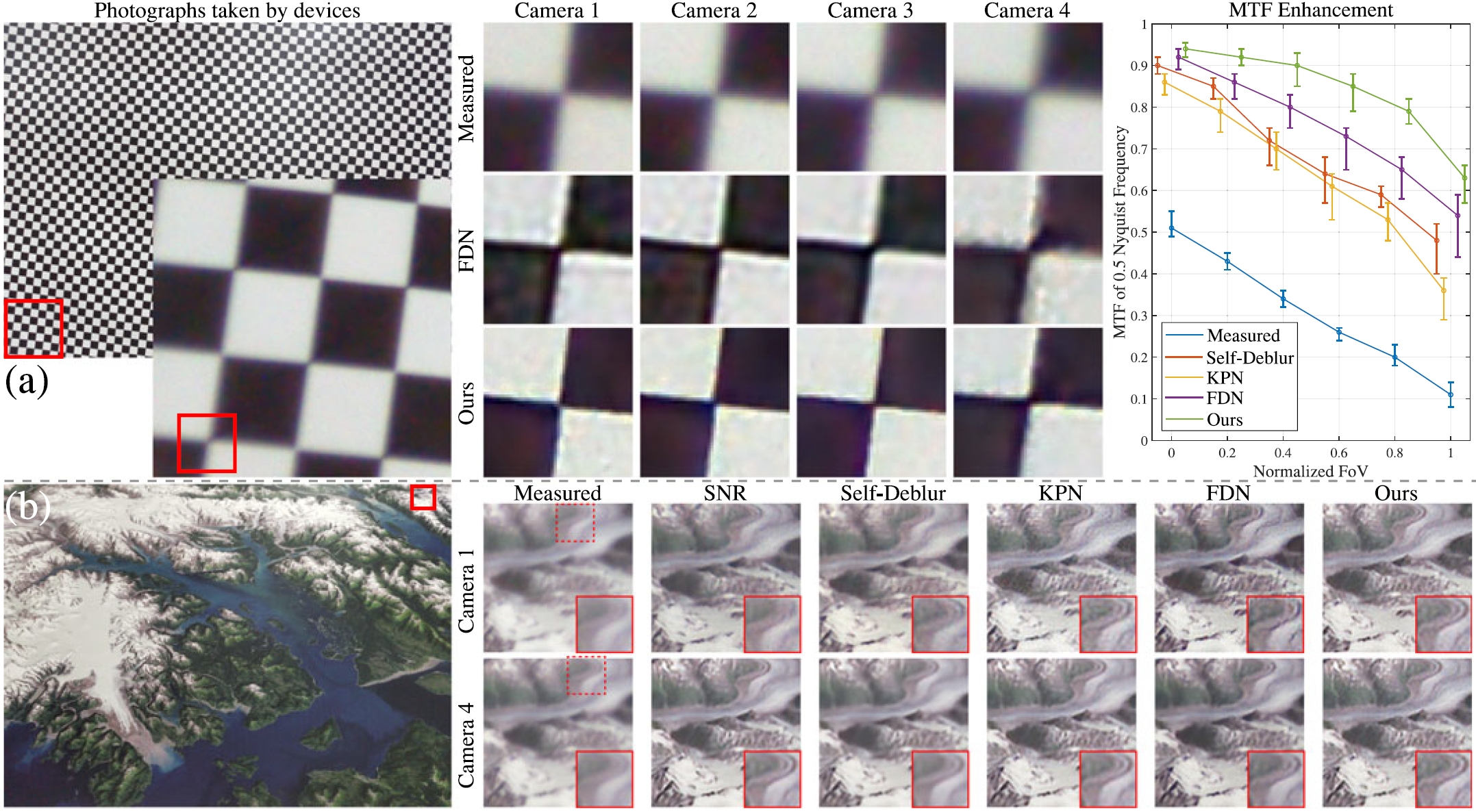
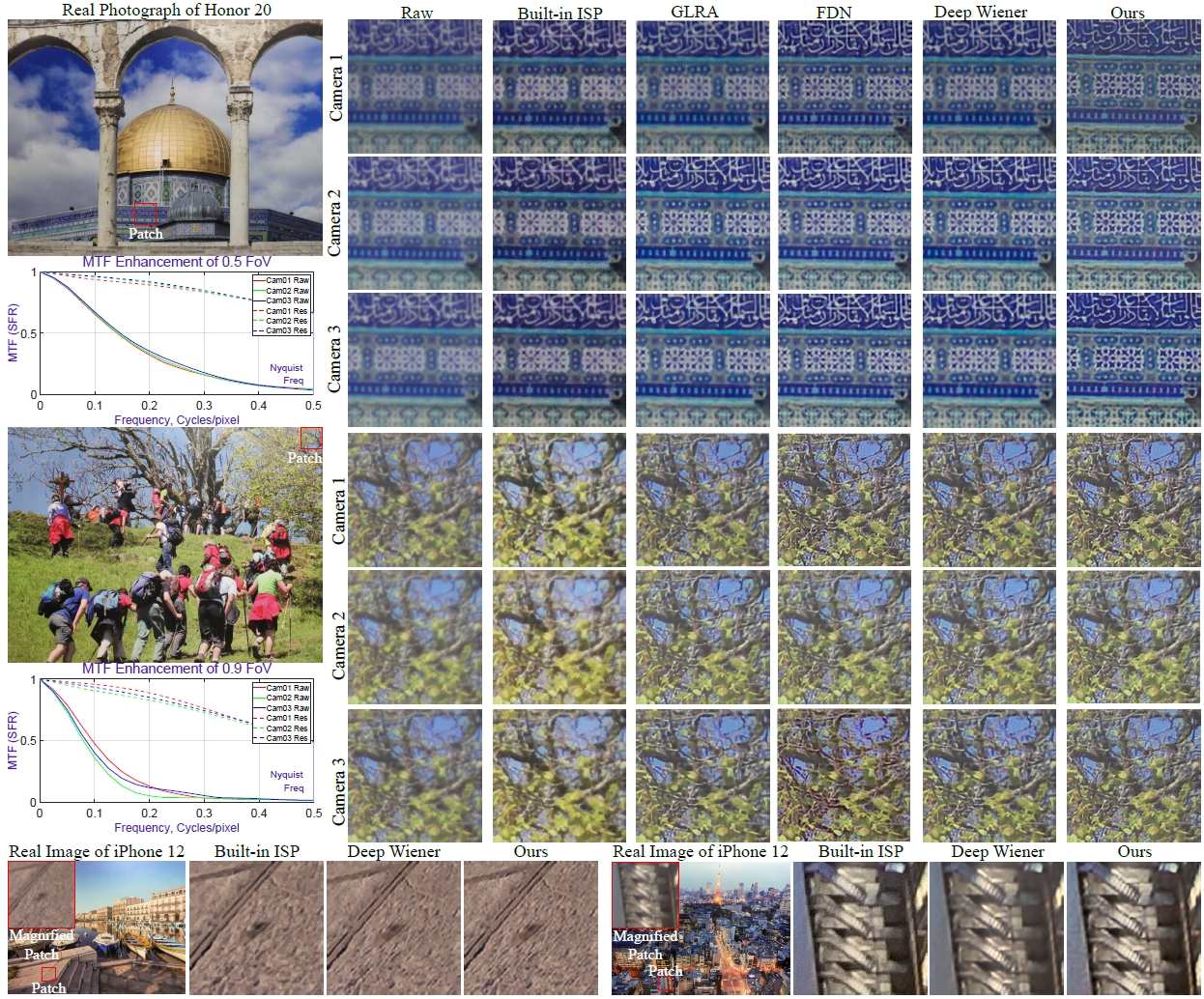
We also present an interesting ablation here, evaluating how the different optical prior influence the restoration.
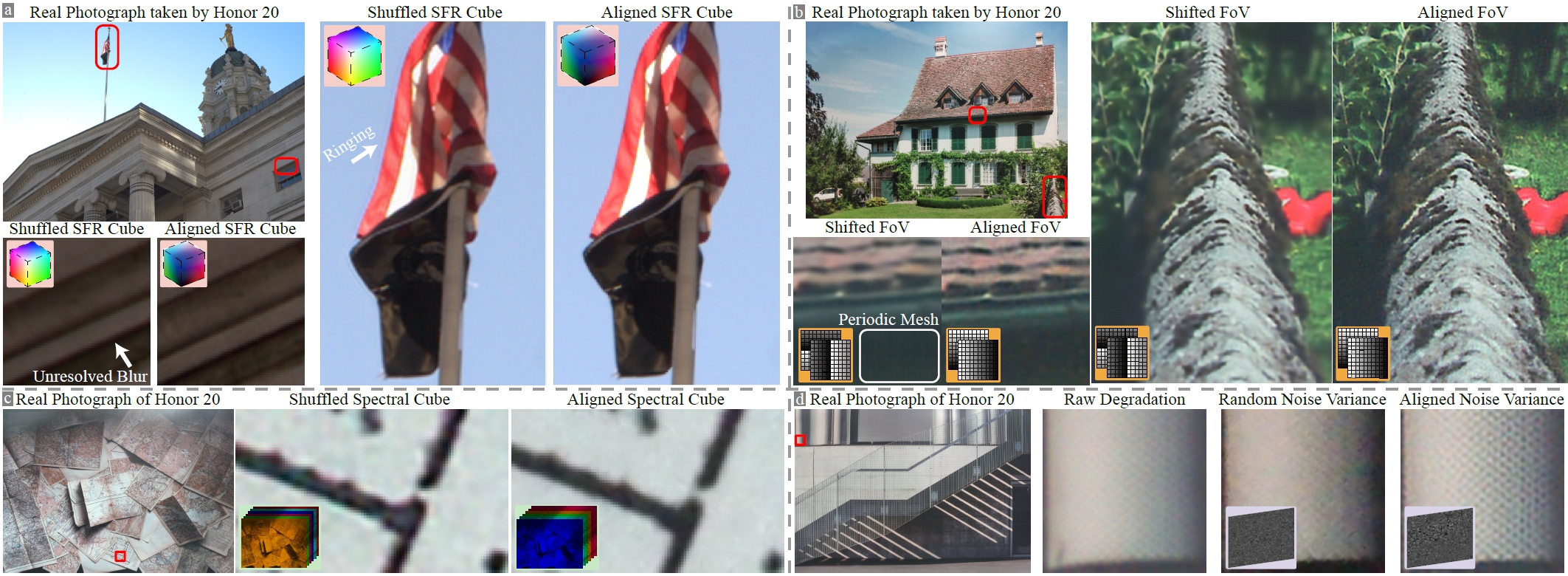
The benifits to the down-stream application:
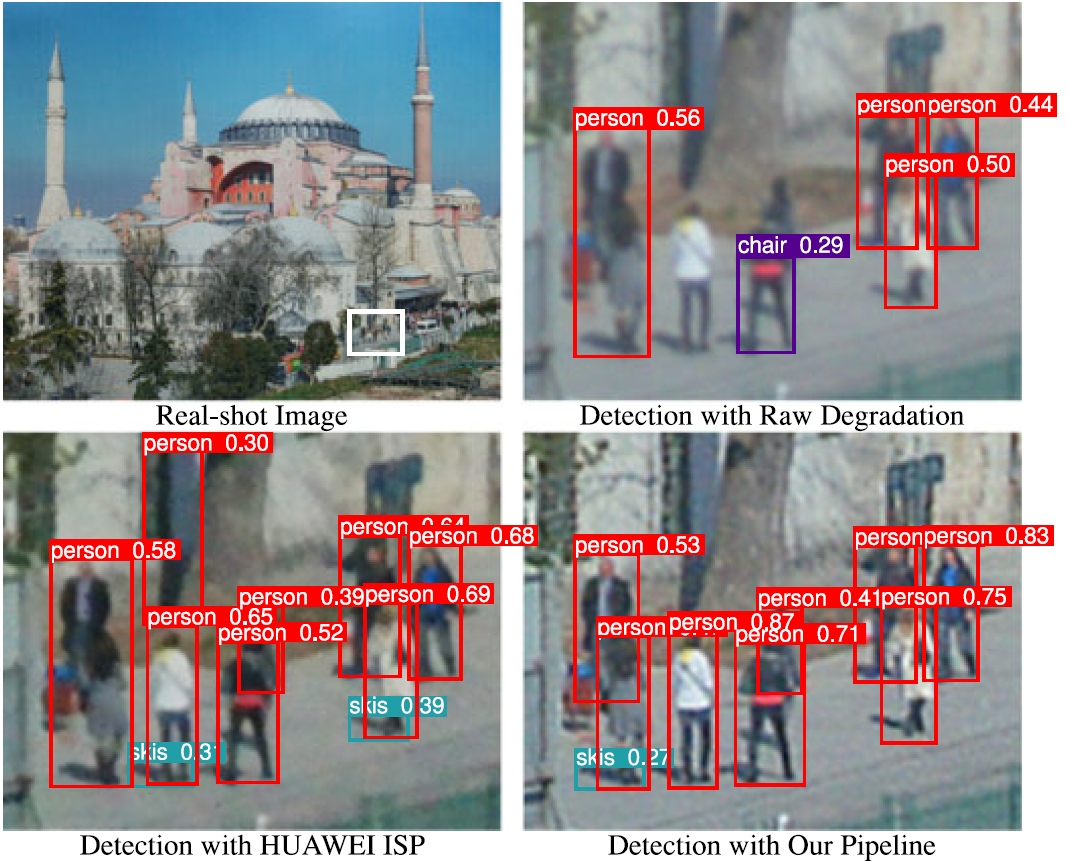
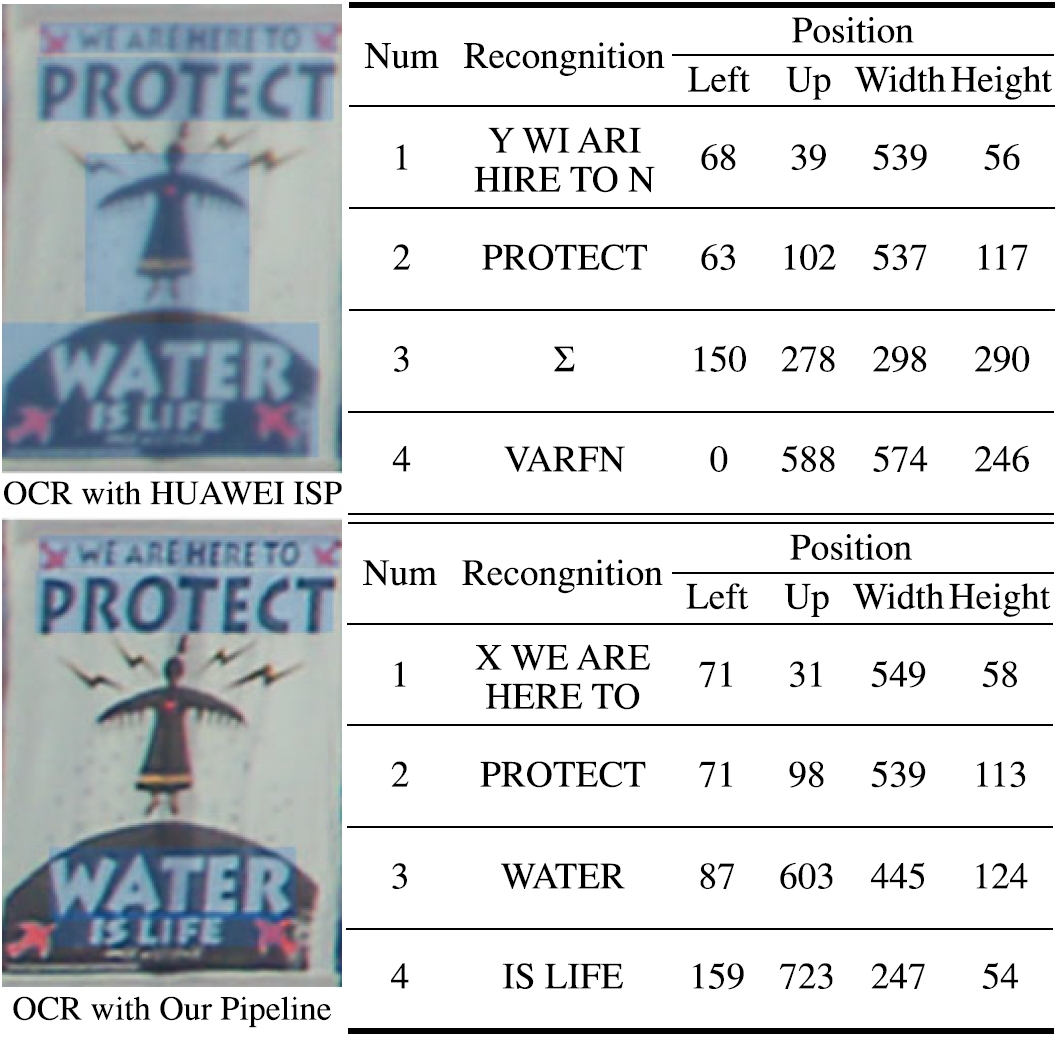
Our Main Observation:
- With the proxy cameras from multiple machining samples, we synthesized the data pairs with complex degenerate distributions, aiming at encoding the optical aberrations and the random bias introduced during processing.
- It is convenient to deploy the proposed model in the mass production of arbitrary imaging devices. And this work has been applied to some mobile terminals to realize significantly improved imaging.